The Role of AI and Automation in Product Engineering
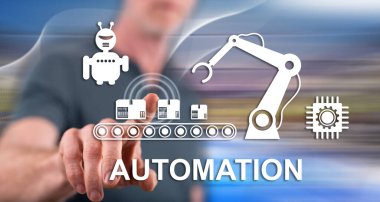
In today’s rapidly evolving digital landscape, the integration of Artificial Intelligence (AI) and Automation is revolutionizing the way products are designed, developed, and delivered. AI-driven solutions and automation tools offer immense potential to optimize product engineering processes, improve efficiency, and enhance the quality of outputs. Companies that adopt AI in their engineering workflows can innovate faster, reduce costs, and stay competitive.
In this blog, we’ll explore the critical role of AI and automation in product engineering and delve into specific use cases, including predictive maintenance, AI-driven testing, and how automation enhances quality assurance.
1. Predictive Maintenance for Reduced Downtime:
One of the most impactful use cases of AI in product engineering is predictive maintenance, which leverages machine learning (ML) models to forecast when equipment or systems might fail, thus preventing costly downtimes. By analyzing data from sensors and IoT devices, AI can predict maintenance needs before a failure occurs, leading to proactive action rather than reactive fixes.
Use Case in Manufacturing: In industries such as automotive or aerospace manufacturing, AI-powered predictive maintenance helps monitor the health of machinery in real-time. General Electric (GE) uses AI to monitor its jet engines and turbines, predicting potential failures and optimizing the maintenance schedule to reduce unscheduled downtimes, which leads to significant cost savings.
IoT and AI Integration: Combining Internet of Things (IoT) devices with AI systems helps companies gather vast amounts of operational data. This data is then processed by machine learning algorithms to identify patterns that signal potential breakdowns, enabling timely interventions.
2. AI-Driven Testing for Faster, Accurate Results
Traditional testing methods in product engineering are time-consuming and prone to human error. AI-driven testing offers a solution by automating the test process and making it more efficient. With AI, testing cycles are shortened, and bugs or issues can be identified earlier in the development phase.
Automated Code Testing: AI tools such as Testim and Functionize can automatically create, execute, and adapt tests for software products. These AI-driven platforms use machine learning to analyze test data and continuously refine test cases, ensuring that quality remains high throughout the development cycle.
Regression Testing: AI algorithms can execute regression tests on large-scale software systems, ensuring that new changes don’t disrupt existing functionality. AI-driven testing tools are especially useful for applications that require frequent updates, as they enable faster iterations without sacrificing quality.
Visual Testing for UI/UX: AI also plays a critical role in UI/UX testing, where it can automatically detect visual discrepancies in user interfaces across different devices or browsers. For instance, AI tools can ensure that changes in code don’t negatively impact user experience across platforms.
3. Automation in Quality Assurance: Enhancing Accuracy and Speed
In the product engineering process, maintaining high standards of quality is non-negotiable. Traditional quality assurance (QA) methods can be resource-intensive, requiring manual checks that are often slow and prone to error. By introducing automation into the QA process, companies can ensure faster testing, more consistent results, and greater reliability.
Automated Defect Detection: AI-powered tools can analyze large datasets to detect patterns and identify defects in real time. For example, in electronics manufacturing, automated systems can inspect printed circuit boards (PCBs) using image recognition technology to quickly detect defects that human inspectors might miss.
AI for Automated Bug Fixing: Machine learning algorithms are increasingly being used to automatically detect, diagnose, and even fix bugs. Facebook’s SapFix, for example, is an AI system that identifies bugs and suggests potential fixes, speeding up the bug resolution process significantly.
End-to-End Automation with DevOps: Integrating AI-driven QA into the DevOps pipeline ensures continuous integration and continuous deployment (CI/CD) with automated testing at each stage. This minimizes human intervention and makes the QA process faster and more accurate.
4. Machine Learning for Product Design and Development
Beyond testing and maintenance, AI is playing a pivotal role in the design and development phases of product engineering. With AI-driven insights, companies can optimize their products by analyzing user data, performance metrics, and environmental factors, resulting in more refined and tailored products.
Generative Design: AI can automatically generate multiple design variations based on predefined parameters. Autodesk’s Dreamcatcher is an example of a generative design tool that uses machine learning to create design options optimized for weight, material, and performance, allowing engineers to select the best option from thousands of AI-generated solutions.
Customer-Centric Design: By analyzing vast amounts of user data, AI can provide insights into customer preferences and behaviors. These insights can inform the development of more user-centric products, ensuring that they meet market needs more precisely.
5. Accelerating Time to Market with AI
AI not only optimizes processes but also reduces time to market, helping companies launch products faster without compromising on quality. By automating repetitive tasks such as data analysis, bug testing, and even parts of the design process, AI frees up engineers to focus on innovation and more complex problem-solving tasks.
AI in Prototyping: Using AI-driven simulations, product teams can quickly test prototypes and gather feedback without building physical models. This accelerates the iteration process, ensuring that products are refined and ready for market faster.
AI in Resource Allocation: Machine learning models can also predict resource needs for engineering projects, ensuring that the right amount of resources (time, materials, labor) is allocated for each phase. This predictive capability reduces project delays and optimizes time-to-market strategies.
6. AI in Supply Chain Optimization
A crucial part of product engineering is ensuring that the supply chain is well-managed and efficient. AI-powered supply chain solutions enable companies to optimize inventory levels, forecast demand, and identify potential bottlenecks.
Inventory Management: AI tools can analyze historical data and market trends to predict demand accurately. Amazon, for instance, uses AI to optimize its inventory management and logistics, ensuring that products are available when needed without overstocking.
Logistics and Distribution: AI-driven analytics can also optimize logistics, reducing shipping times and ensuring timely delivery of products. This capability is especially crucial for businesses looking to reduce time to market and improve customer satisfaction.
Conclusion
The role of AI and automation in product engineering is transformative. From predictive maintenance to AI-driven testing and automated quality assurance, these technologies are reshaping how products are developed, tested, and delivered. Companies that embrace AI and automation can not only accelerate their product development cycles but also ensure higher quality, better customer experiences, and optimized resource management.
At Raphus Solutions,we specialize in helping businesses integrate AI and automation into their product engineering processes, enabling them to innovate faster, reduce costs, and deliver superior products to market.
By adopting AI-driven solutions, companies can stay competitive in today’s fast-paced market, ensuring that their products are always ahead of the curve.